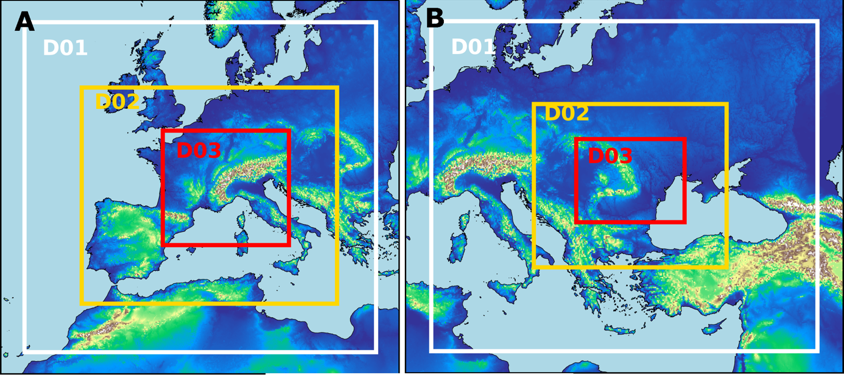
MAGDA: Redefining Precision Forecasting with Cutting-Edge Technology
Explore the future of agricultural innovation with MAGDA. We strive to enhance weather forecasting by integrating real-time data to assist farmers in making informed decisions. From predicting weather patterns to adapting to evolving conditions, MAGDA provides tools to optimise agricultural practices and ensure a sustainable future.
At the heart of MAGDA’s forecasting system lies the Weather Research and Forecasting (WRF) model, meticulously configured to cover Italian, French, and Romanian sites. Utilising two domain configurations, each setup includes three nested domains with resolutions ranging from 22.5 to 2.5 kilometres (Figure 2). These configurations, coupled with data assimilation techniques, enable MAGDA to capture fine-scale atmospheric phenomena with good precision (Figure 1).
MAGDA’s forecasting system leverages data assimilation to seamlessly integrate observational data into model simulations, refining forecast accuracy. By assimilating diverse observations, including radar data, ground stations, GNSS data, and drone-borne measurements, MAGDA ensures comprehensive coverage and real-time updates. Through specific algorithms, MAGDA optimises the assimilation process, enhancing forecast reliability across various applications. The various types of observational data are integrated into the WRF model after different post processing steps:
- Radar Data: Converted and thinned to match model resolution. Different regions have specific procedures for converting radar data into a format compatible with the WRF model.
- GNSS Data: Processed using scripts to convert data into the proper format for assimilation.
- Temperature Data: Converted from CSV format to the required ASCII format for WRF ground stations assimilation.
- Meteodrone Data: High-resolution vertical profiles re-gridded to match model levels and converted for assimilation, they will be used in a similar way like soundings are usually used.
Understanding the diverse needs of end-users, MAGDA project tailors forecast timings to specific applications. For hydrological and irrigation purposes, simulations will be a 120-hour forecast, integrating observations at strategic intervals to enhance precision in the initialization. Conversely, for convective storm forecasting, a dynamic approach is adopted, employing more frequent model runs with assimilation of observations closer to the event to accurately predict localized convection phenomena (Figure 1 is an example of hailstorm forecasting).
MAGDA’s forecasting system will undergo rigorous performance analysis and validation to ensure reliability and accuracy. Through comprehensive assessments of assimilation behaviour and model performance, in MAGDA project the methodologies are refine and tailored following the agriculture requirements. While the full validation results will be presented in the future at the end of the work, early tests demonstrate the effectiveness and reliability of MAGDA’s forecasting system across diverse scenarios: Figure 3 is an example of a first object comparison between simulation with and without data assimilation and observations to assess the model performances and the assimilation effect on the forecast. The results from the initial case studies used for setting up the forecasting system have shown that when observations are assimilated with a timing that allows new information to be integrated into the model, the forecast improves, demonstrating that the chosen assimilation approach for different types of observations is correct and behaves as expected.
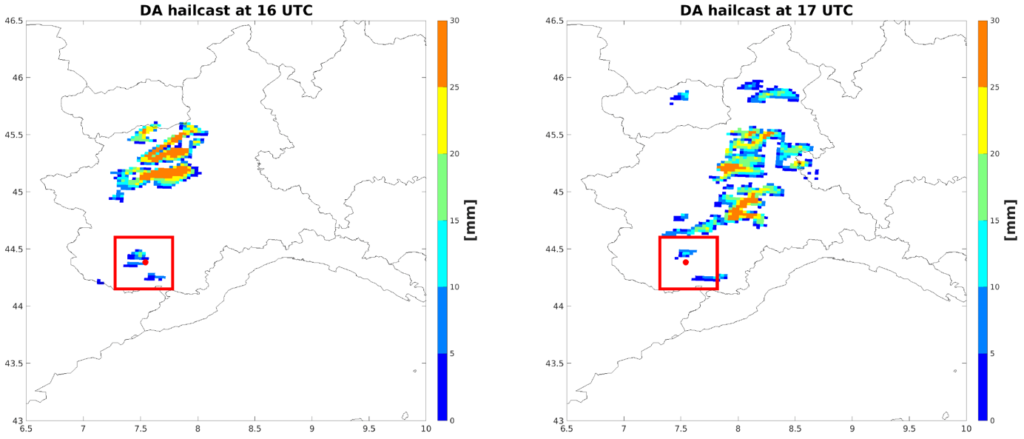
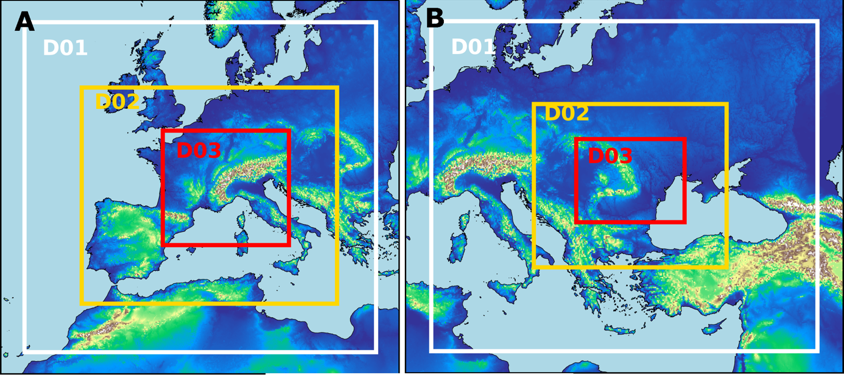
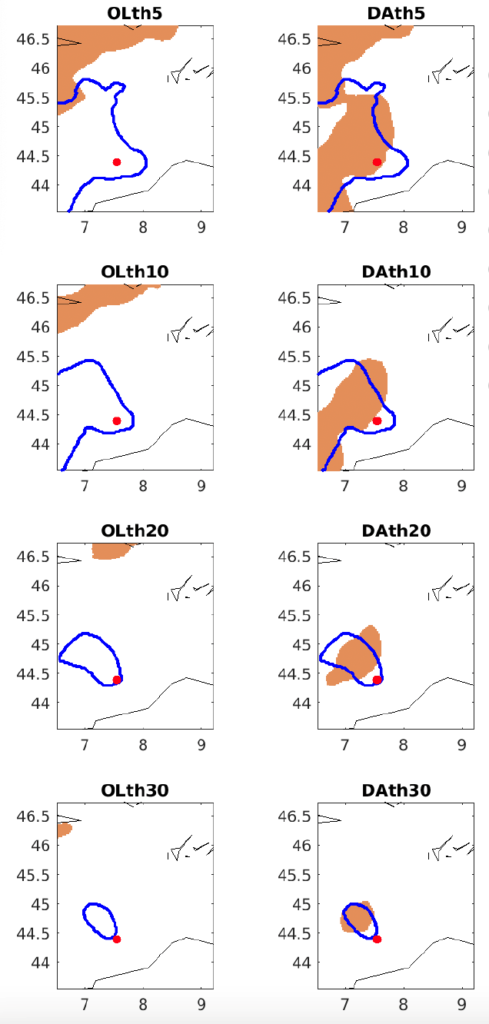