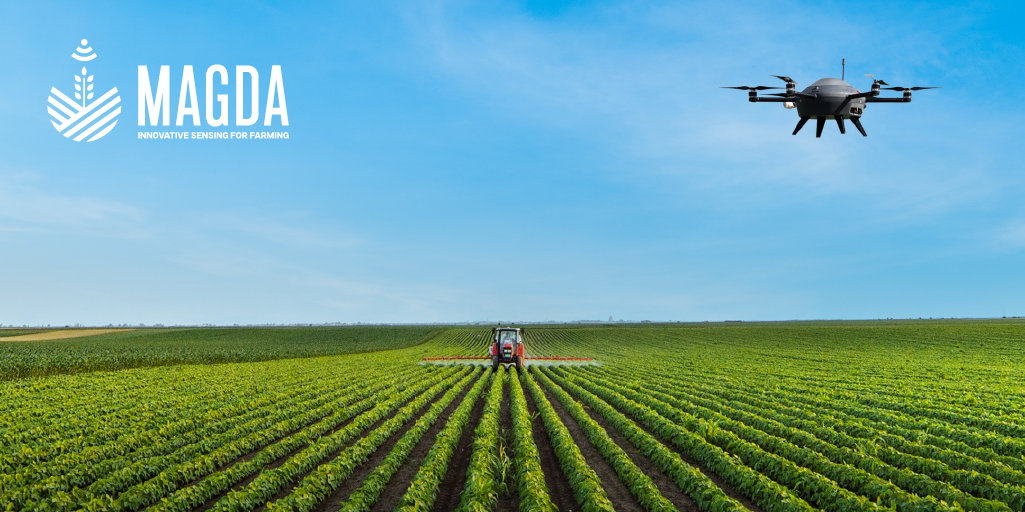
Enhancing Agricultural Weather Forecasts through Vertical Profile Data Assimilation from Meteo-Drones
MAGDA aims to improve weather forecasts for agriculture by using advanced data sources, including weather drones. Our goal is to provide farmers with reliable predictions to adapt to changing conditions, optimize their practices, and support sustainable farming.
MAGDA’s weather forecasting system is designed to enhance agricultural weather predictions by integrating advanced observational data into the Weather Research and Forecasting (WRF) model. A key feature of this system is the assimilation of vertical profiles of atmospheric conditions, which provide detailed measurements of temperature, humidity, wind speed, and other variables at various altitudes. These vertical profiles are crucial for accurately modeling the vertical structure of the atmosphere, which in turn has a significant impact on weather phenomena such as precipitation patterns, storm development, and temperature fluctuations.
In traditional weather forecasting, the vertical structure of the atmosphere is often inferred from ground-based observations or limited balloon soundings, which may not capture the full range of atmospheric conditions, especially in specific regions. By incorporating high-resolution vertical profiles directly from the field, MAGDA improves the accuracy of its forecasts. The data collected by drones, which fly over agricultural fields, provides localized atmospheric information that is directly relevant to the crops and farming practices in that area. This makes the forecasts more precise and tailored to the specific needs of farmers.
The drone-based vertical profiles are processed and re-gridded to match the levels of the WRF model, a process similar to how traditional weather soundings are assimilated into the model. This allows the system to integrate these vertical measurements seamlessly, enhancing the model’s ability to predict localized weather events such as temperature inversions, humidity changes, and convective storms. These localized weather phenomena are particularly important for agricultural applications, where even small variations in atmospheric conditions can significantly affect crop growth, irrigation needs, and pest control.
MAGDA’s data assimilation techniques go beyond vertical profiles, incorporating a range of observational data, including radar, GNSS, and ground station measurements. This multi-source approach ensures comprehensive coverage of the atmosphere and allows for real-time updates to the forecast. The system tailors its forecast timings and assimilation strategies based on the specific needs of the agricultural sector. For example, for hydrological and irrigation purposes, MAGDA produces 120-hour forecasts with strategic integration of observational data at key intervals to improve initialization precision. In contrast, for convective storm forecasting, the system runs more frequent model updates and assimilates observations closer to the event to accurately predict localized convective phenomena, such as hailstorms.
The drone assimilation tests demonstrated that the assimilation method successfully brings the model closer to the observations, providing a better representation of the vertical atmospheric profile, particularly in the lower layers, at the location of the cultivated field of interest. Figure 1 illustrates this with an example showing how the temperature profile changes between the background (blue line) and the forecast (red line in the left panel). After assimilation, the analysis (red line in the right panel) aligns much more closely with the observation (black line), especially in the lower atmospheric layers.
Early tests of the MAGDA system demonstrated that assimilating these profiles, along with other observational data, results in more reliable and precise weather forecasts. This approach is the first assessment about the importance of using vertical profile data to enhance weather predictions, especially in agricultural contexts where timely and accurate weather information is essential for optimizing crop management and ensuring a sustainable farming future.
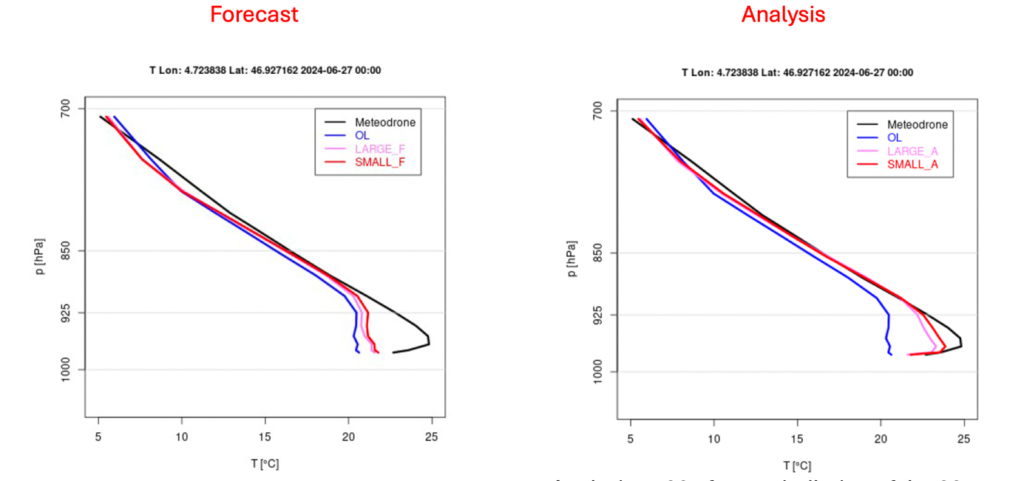