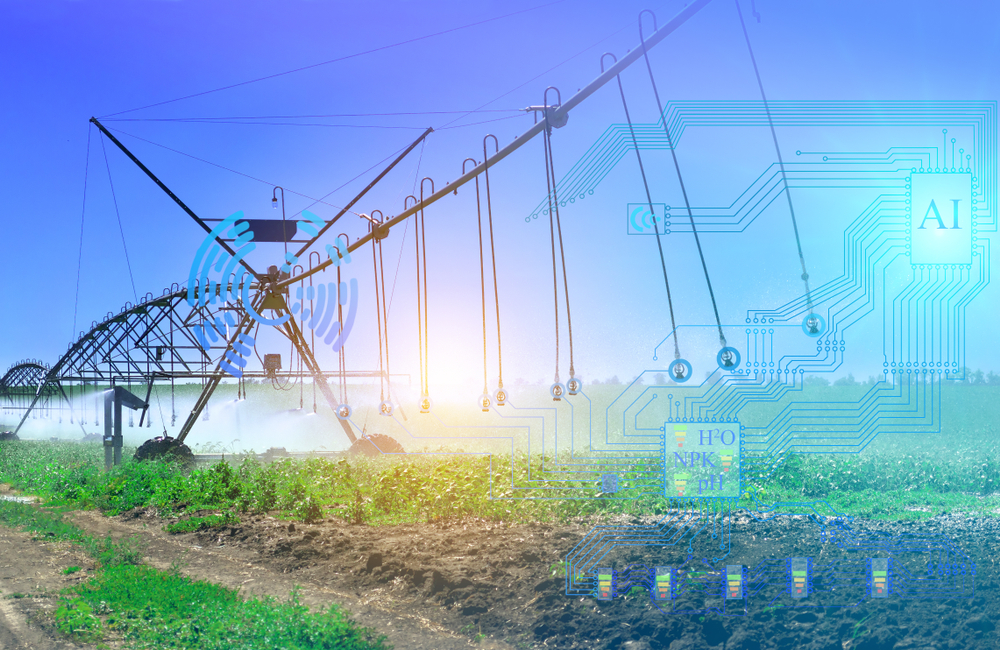
Machine Learning: The Future of Smart Irrigation
Cover image: KoSSSmoSSS/Shuttertsock.com
A recent study highlights how Machine Learning (ML) is transforming smart irrigation, offering efficient water management solutions for agriculture. Researchers from Abdelmalek Essaadi University explored how ML models enhance evapotranspiration (ET) prediction and irrigation scheduling, helping to optimize water use in the face of climate challenges.
With agriculture consuming 70% of global freshwater, traditional irrigation methods often lead to waste. Smart irrigation systems, powered by real-time sensors and ML algorithms, dynamically adjust water distribution based on weather patterns, soil moisture, and crop needs, ensuring efficiency and sustainability.
The study highlights ML models like Random Forest, Support Vector Machines, Artificial Neural Networks, and XGBoost, which outperform traditional methods in ET estimation. These models process large datasets, providing precise irrigation recommendations, reducing water waste, and improving crop yields.
Despite its promise, data availability, model interpretability, and computational demands remain challenges. Future research aims to develop more accessible ML solutions, integrate IoT-based monitoring systems, and enhance climate-resilient irrigation models. Collaboration among policymakers, researchers, and farmers will be essential to maximize adoption.
As climate change intensifies, ML-powered irrigation offers a sustainable path forward, ensuring farmers can optimize water use while maintaining productivity. The future of agriculture is smart, data-driven, and AI-powered.
The whole article can be found here:
https://www.e3s-conferences.org/articles/e3sconf/pdf/2025/01/e3sconf_icegc2024_00078.pdf
A Review on Optimizing Water Management in Agriculture through Smart Irrigation Systems and Machine Learning
Zaid Belarbi, Yacine El Younoussi
E3S Web Conf. 601 00078 (2025)
DOI: 10.1051/e3sconf/202560100078
Author: Amelia Fernández Rodríguez
Keywords
irrigation timing and amounts; surface soil moisture; data assimilation; particle filters; FAO-56; Sentinel-1; semi-arid Mediterranean region; winter wheat