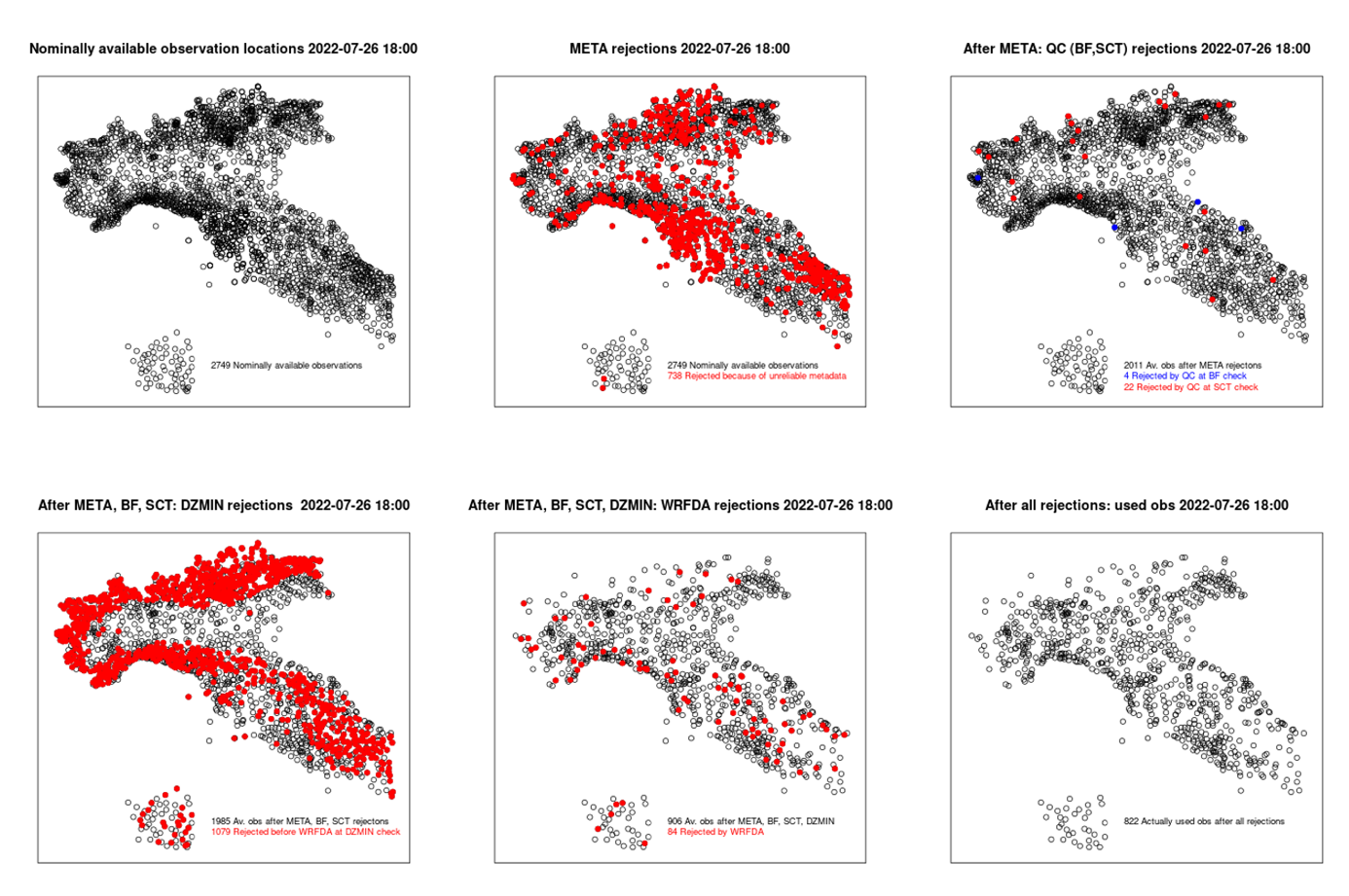
Automatic quality control checks enable the use of dense observational networks in convection-permitting simulations
The MAGDA project aims to improve weather forecasts by assimilating high-resolution data from sources like GNSS, weather radars, drones, and ground-based stations. However, these data can contain errors that negatively affect the models. The CIMA weather group developed automatic quality control (QC) checks to detect such errors, with initial experiments focusing on Italian temperature stations. Results showed that assimilating these observations impacted simulations, paving the way for future forecast improvements. QC checks were crucial in ensuring data consistency, and further experiments with other data sources will help assess their potential for enhancing weather forecasts.
One of the aims of the MAGDA project is to enhance weather forecasts by assimilating data from various types of high-resolution observations such as GNSS (Global Navigation Satellite System), weather radar data, meteorological drones and in situ observations from ground-based stations. With regard to this last source of meteorological information, for each country involved in the MAGDA use cases, there are official authoritative networks, low-cost networks and sensors that have been installed during the project. All these source of data can present different type of errors, that can negatively affect the model forecast if they are assimilated as they come. These kind of data from such high-resolutions networks require careful checks to detect gross errors, always present in such observational systems. In consideration of the large number of observations and of the operational time constraint, Quality Control (QC) checks must necessarily be automatic. Moreover, a periodic check of basic station metadata is necessary, to detect errors, imprecisions and unreported changes in information on station locations: geographical coordinates and elevation above mean sea level.
At the weather group of CIMA, the ongoing activity on QC check of weather observations has been developed also in the MAGDA framework starting from the Italian use case (Figure 1). The Italian National Civil Protection network collects thousands of observations every hour, covering all Italy and providing detailed and valuable information on meteorological fields. This enabled testing the use of temperature observations from ground-based stations in assimilation experiments with the high-resolution, convection permitting model WRF, in the same configuration used for numerical weather forecasts in the context of MAGDA. The QC checks make use of advanced algorithms based on the concept of spatial consistency among neighbouring stations.
The experiments, in which only in situ temperature observations were assimilated to enhance their impact (if any) showed two main results.
First, that assimilating such observations did in effect have an impact on the simulation, with respect to a free evolution run produced for comparison, and that it lasted for several forecast hours after the data assimilation time period. This is a non-trivial result, meaning that the signal from ground stations was consistent with the model dynamics. This did not result in a forecast improvement, which was not expected at this stage: it means that a forecast improvement is possible. Further experiments making also use of other types of observations, such as weather radar reflectivity, will help assessing that.
Moreover, the impact of QC checks was effective in making the 1-hour forecast more consistent with the new observation set (to be assimilated at the next analysis time) when that forecast resulted from a (previous) analysis state obtained by assimilating observations that underwent the QC check, with respect to a control run in which the “raw” dataset was fed to the assimilation procedure without undergoing the QC tests (Figure 2). Differences between the two assimilations runs, with QC and without QC, also lasted for several forecast hours after the last analysis time.
This first evaluation of the QC procedure has been done on an authoritative network, as it should be the most reliable data provider. This work paves the way to integrate into the model also low-cost sensors information avoiding the risk of providing large errors to the model that can negatively affect the simulation instead of improving it.
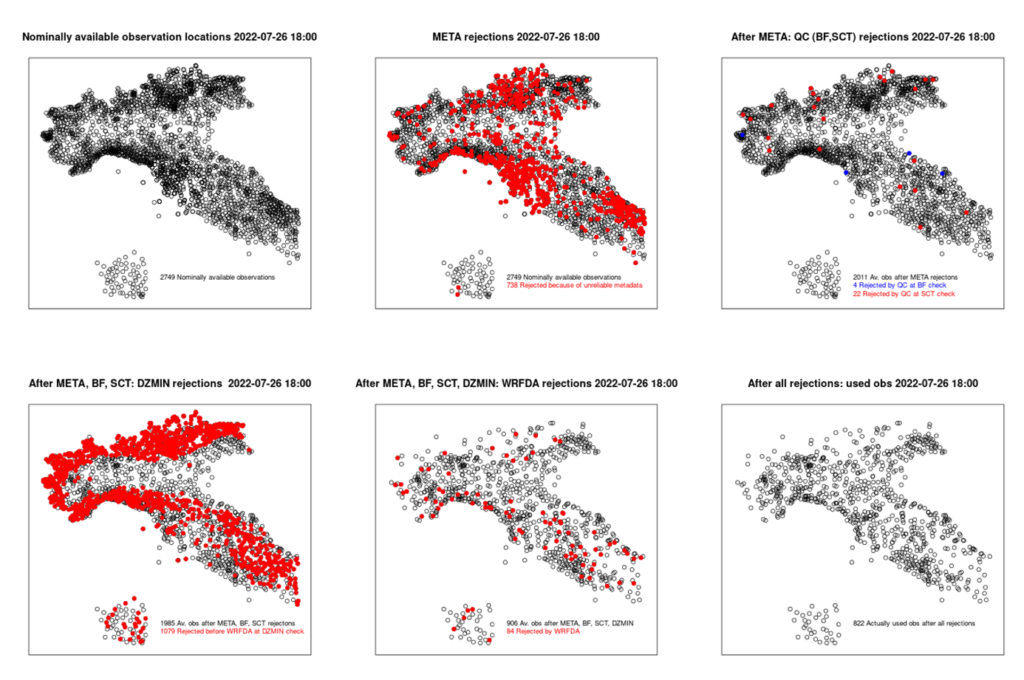
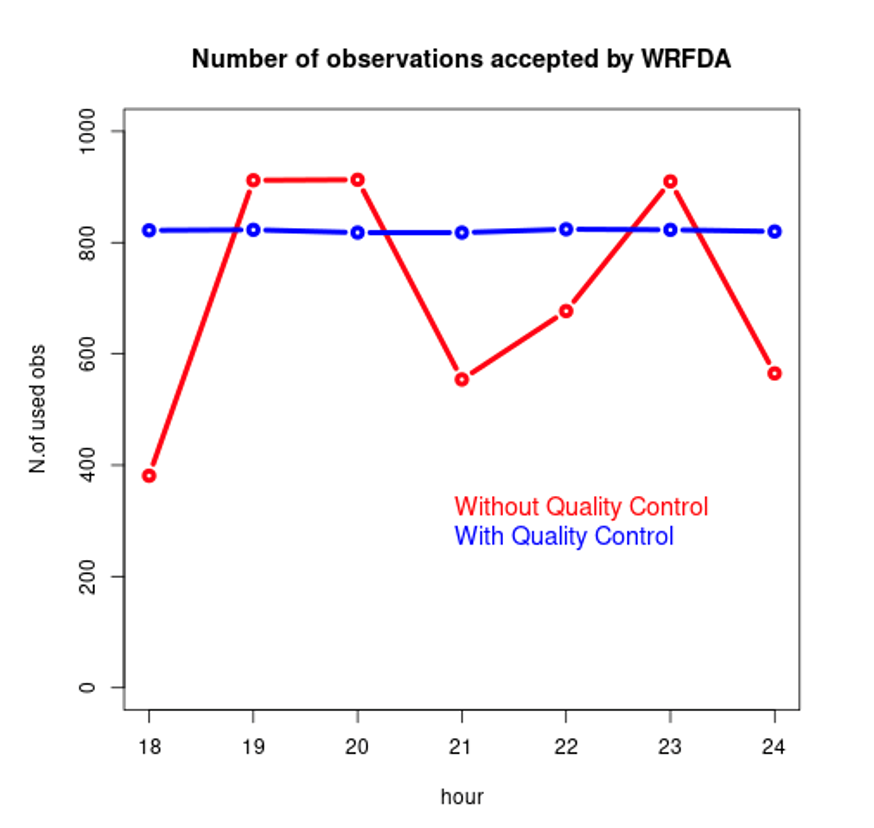