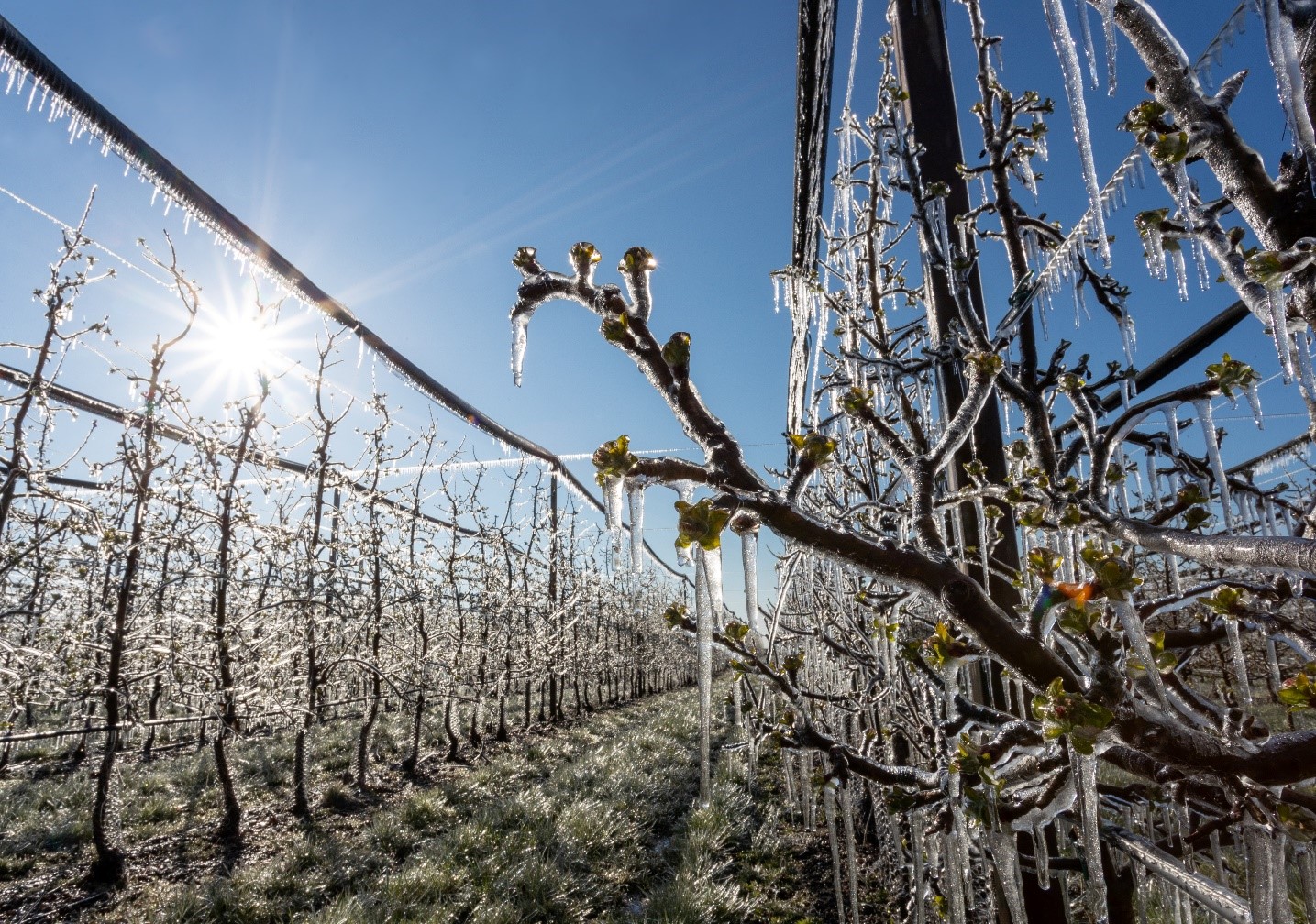
Agriculture in a Changing Climate: Data Assimilation in NWP Models as a Key Tool
Agriculture faces significant challenges due to the impacts of climate change. Rising temperatures, altered precipitation patterns, and more frequent extreme weather events impact crop production. In this context, data assimilation techniques in Numerical Weather Prediction (NWP) models become an important tool. By integrating real-time observations into forecasts, data assimilation enhances accuracy, empowering farmers to adapt to climate change. This article explores the agricultural issues related to climate change, highlighting the importance of data assimilation in NWP models to improve forecasts of extreme events, and discusses potential outcomes that can benefit agriculture.
Why Climate Change has an impact on agriculture:
Climate change alters weather patterns, resulting in adverse effects on agriculture. Increased temperatures disrupt crop growth, changes in precipitation patterns lead to droughts or floods, and extreme weather events like hurricanes, storms, hailstorms, and late freezes damage crops, infrastructure, and livestock. Understanding and forecasting these events is key to implementing effective mitigation and adaptation measures in agriculture. Hailstorms cause physical damage to crops[1][2], reducing photosynthetic capacity and increasing vulnerability to secondary infections. Rainfall patterns influence pest and fungal disease prevalence, affecting crop health [3]. Late freezes harm crops by causing frost damage to flowers, buds, and young fruits and also leading to reduced yields due to lacking pollination. Understanding these impacts helps farmers implement protective measures and adjust farming practices.
Numerical Weather Prediction (NWP) models and data assimilation:
Numerical Weather Prediction (NWP) models simulate and predict weather patterns by utilizing complex mathematical equations based on atmospheric physics. These models are powerful tools in understanding and forecasting weather conditions evolution in time and space. However, to further improve their accuracy and reliability, data assimilation plays a crucial role. In fact, data assimilation acts as a bridge between observed data and the mathematical equations used in NWP models. By integrating real-time observations from various sources, such as weather stations, radars, and satellites, it helps correct any errors or biases in the initial model conditions, enhancing the model’s ability to generate accurate and reliable forecasts [4]. By continuously assimilating real-time observations, NWP models can update and refine their forecasts, providing improved predictions of temperature, precipitation, wind patterns, and other meteorological variables relevant also for agricultural issues such as the probability and the expected diameter of hail. Accurate forecasts can empower farmers to take proactive measures, such as adjusting planting schedules, optimizing irrigation practices, and implementing appropriate pest and disease management strategies.
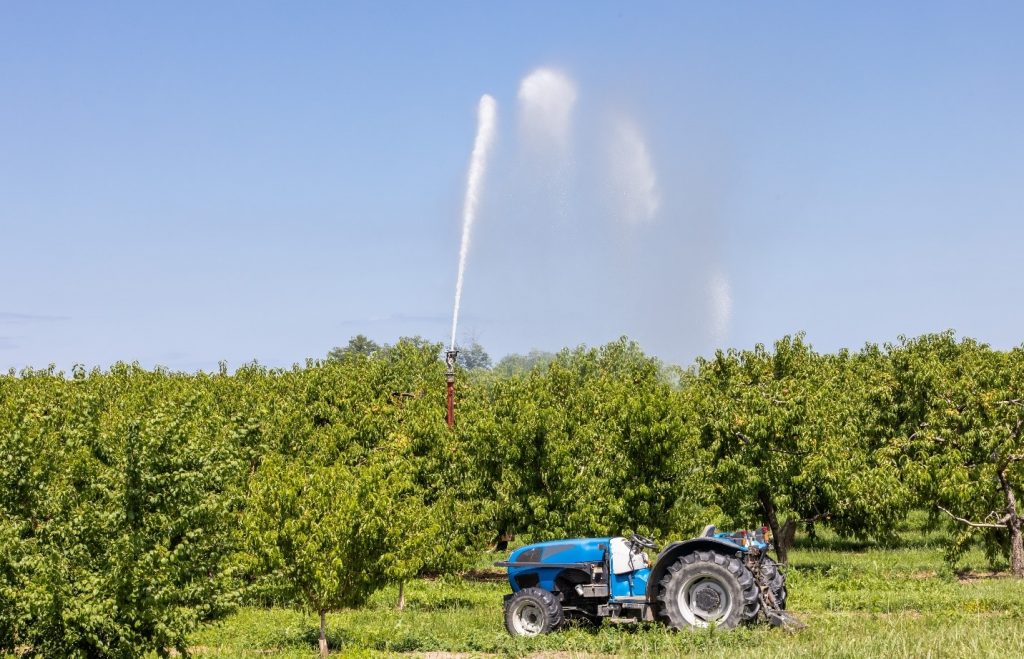
Harnessing the power of improved meteorological forecasts in agriculture:
Accurate meteorological forecasts can play an important role in supporting agricultural resilience through optimized water management, improved pest and disease control, and proactive measures against severe weather events, including severe storms, extreme temperatures, hailstorms, and late freezes. By providing precise predictions coupled with hydrological and irrigation model outputs, farmers can optimize irrigation practices, allocate water resources efficiently, and manage reservoirs effectively, resulting in improved water-use efficiency.
Integrated with pest and disease models, accurate forecasts allow for proactive measures such as timely pesticide applications, adjusted planting schedules, and integrated pest management strategies, reducing crop losses. Additionally, advanced warnings of damaging-producing weather systems allow farmers to implement protective measures, mitigating the economic losses. By harnessing more accurate meteorological forecasts, agriculture can strengthen resilience, promote sustainable practices, and better facing the ongoing changing climate.
Authors Martina Lagasio, Vincenzo Mazzarella, Massimo Milelli, Antonio Parodi
References
[1] Kleynen, V. Leemans, M.F. Destain (2005) Development of a multi-spectral vision system for the detection of defects on apples. Journal of Food Engineering 69 (1), 41-49.
[2] T. Riquelme, P. Barreiro, M. Ruiz-Altisent, C. Valero (2008) Olive classification according to external damage using image analysis. Journal of Food Engineering 87 (3), 371-379.
[3] Simon, G. (2006) Review on rain induced fruit cracking of sweet cherries (Prunus avium L.), its causes and the possibilities of prevention. International Journal of Horticultural Science, 12(3), 27-35.
[4] Lagasio, M.; Silvestro, F.; Campo, L.; Parodi, A. (2019) Predictive capability of a high-resolution hydrometeorological forecasting framework coupling WRF cycling 3dvar and Continuum. J. Hydrometeorol. 20, 1307–1337.
Keywords
Climate change, numerical weather prediction, data assimilation, agriculture, forecast.
Images
Shutterstock